About the project
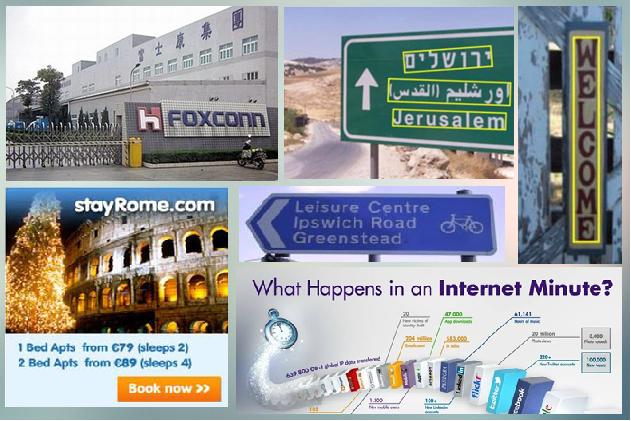
        In this project, we will combine efforts and expertise of two research labs from document analysis community towards achieving the goal of mining and retrieval of weakly structured contents of social networks. Weakly structured – or non-structured -- contents concern specifically a large set of images that can now be found on social networks, which have mostly been captured by mobile devices or synthesized by image editing tools. These image contents can be categorized into four classes: scene images, scanned documents, camera-captured paper documents, and synthesized (born-digital) documents. From these image classes, we mainly consider scene images with embedded text and born-digital documents. Those two image classes are more popular in social networks and bring new technical challenges compared to traditional paper documents. Analyzing the contents of those two image classes will help in the development of the next generation of search engines. Achieving this goal will be very useful for applications like cyber security and commercial data mining, and social applications such as interactive tourists’ guidance.         The research plan of the proposed system is composed of complementary parts that finally form a pipeline of a complete system. First, different image types are received as input; they will be classified by the “fast image categorization” part. Then, scene images will be analyzed by the “scene text detection and extraction” part, whereas born-digital documents will be analyzed by the “layout analysis and graphics recognition” part. The texts extracted from different images types from the previous two parts will be analyzed by the “multi-lingual text recognition” part. Finally, the “conceptual interpretation and information integration” part will combine the information analyzed from the previous parts and integrate them in order to reach a meaningful representation of the document database. The two project partners will collaborate on solving the different problems in accordance with their respective expertise.         The outcomes of this project are on multiple levels. First, from a scientific point of view, this project embodies a strategic move for the L3i and the NLPR labs in the document analysis and recognition community. This project will nourish innovations for our labs and for the community. It will enable them to anticipate major changes in current and future document images, and also to create strong links with other related scientific communities. From a technical point of view, this project will produce a set of integrated techniques in pattern recognition, machine learning and document image analysis fields. This will enable tackling deep knowledge extraction in document stream or in the Web by recovering an important amount of hidden text data. Moreover, the development of large-scale experiments will allow researchers to push their technologies to a new maturity level, and facilitate technology transfer to industrial partners. Finally, from an economical point of view, the technological outcomes of this project could benefit several markets such as advanced web search and social media monitoring for security and safety issues.